Clinical Support for PSY
Published papers in peer-reviewed journals
The clinical validity of using AI with genetic and environmental data to predict response to antidepressants
Objective
To generate an accurate predictor of response to a panel of antidepressants and optimize treatment selection using a data-driven approach analyzing combinations of genetic, clinical, and demographic factors.
Method
State-of-the-art machine learning (ML) tools were employed on the Sequenced Treatment Alternatives to Relieve Depression (STAR*D) study and the Pharmacogenomic Research Network Antidepressant Medication Pharmacogenomic Study (PGRN-AMPS), to generate a predictive algorithm.
To validate the results and confirm the algorithm’s external generalizability outside of its training groups, its capacity to predict individualized antidepressant responses on separate validation and test sets consisting of 622 patients from both studies were assessed.
Results
In the STAR*D patient validation set, a 72.3% average balanced accuracy was achieved across the medications. This compared to 46.8% average initial response rate for unguided treatment selection in the same set of STAR*D study participants. Very similar results were also shown testing an overlapping medication, citalopram, to the external test set of the PGRN-AMPS study. This validated the generalizability of the PREDICTIX design approach to operate on other studies and with real-world data.
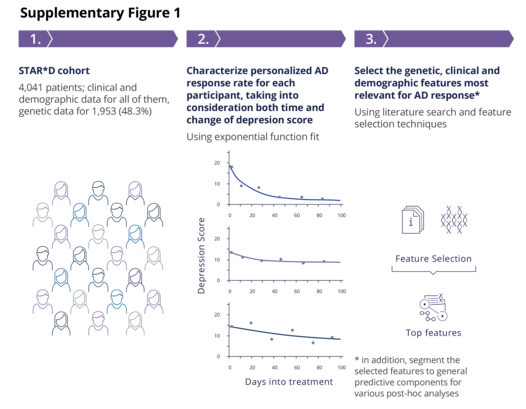
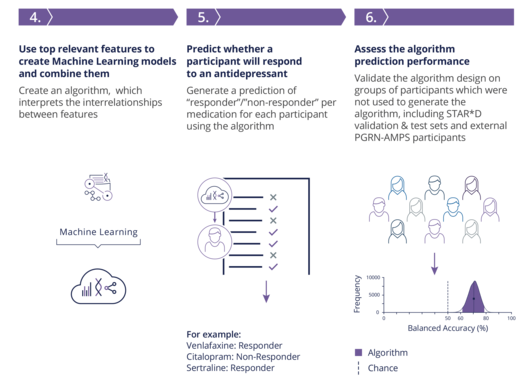
Conclusions
- These results show that using rigorous machine learning approaches and integrated genetic, clinical, and demographic data can help improve prescribing accuracy.
- The use of real-world data will allow us to further improve algorithm performance and apply it to other psychiatric disorders, as well as expand clinical support.
A new characterization of mental health disorders using digital behavioral data: Evidence from major depressive disorder
Background
Mental health disorders are ambiguously defined and diagnosed. The established diagnosis technique, which is based on structured interviews, questionnaires and data subjectively reported by the patients themselves, leaves the mental health field behind other medical areas.
Approach
This paper suggests a paradigm shift is required in which as mental disorders are manifested as behavioural conditions, they therefore should be characterized primarily using behavioral characteristics. We suggest the use of smartphones and wearable devices to continuously and objectively collect quantified behavioral data from patients by utilizing digital biomarkers of mental disorder symptoms. This quantified data can be used to determine a patient’s diagnosis and personalized treatment, and thereby allow a personalized treatment for each patient.
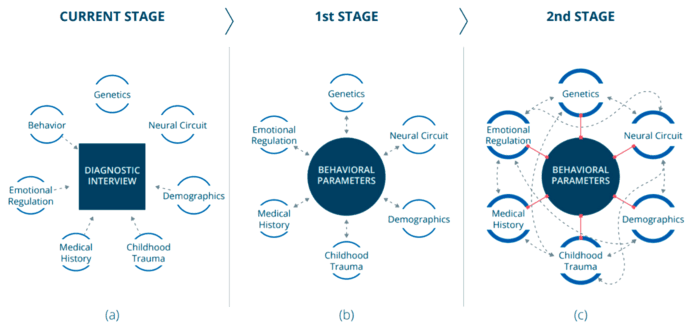
Conclusions
- Since human behavior is a spectrum, such a continuous and personalized approach is essential. By harnessing quantified longitudinal behavioral data, we can unlock the potential of personalized psychiatry. Taliaz is harnessing its scientific-analytical approach to do just that.
The clinical validity of using AI and environmental data to predict response to antidepressants
Objective
To generate an accurate predictor of response to a panel of antidepressants and optimize treatment selection using a data-driven approach analyzing combinations of clinical and demographic factors.
Method
State-of-the-art machine learning (ML) tools were employed on the Sequenced Treatment Alternatives to Relieve Depression (STAR*D) study and the
Pharmacogenomic Research Network Antidepressant Medication Pharmacogenomic Study (PGRN-AMPS), to generate a predictive algorithm. To validate the results and confirm the algorithm’s external generalizability outside of its training groups, its capacity to predict individualized antidepressant responses on separate validation and test sets consisting of 1021 patients from both studies were assessed.
Results
In the STAR*D validation phase, a 64.1% average balanced accuracy was achieved across the medications.
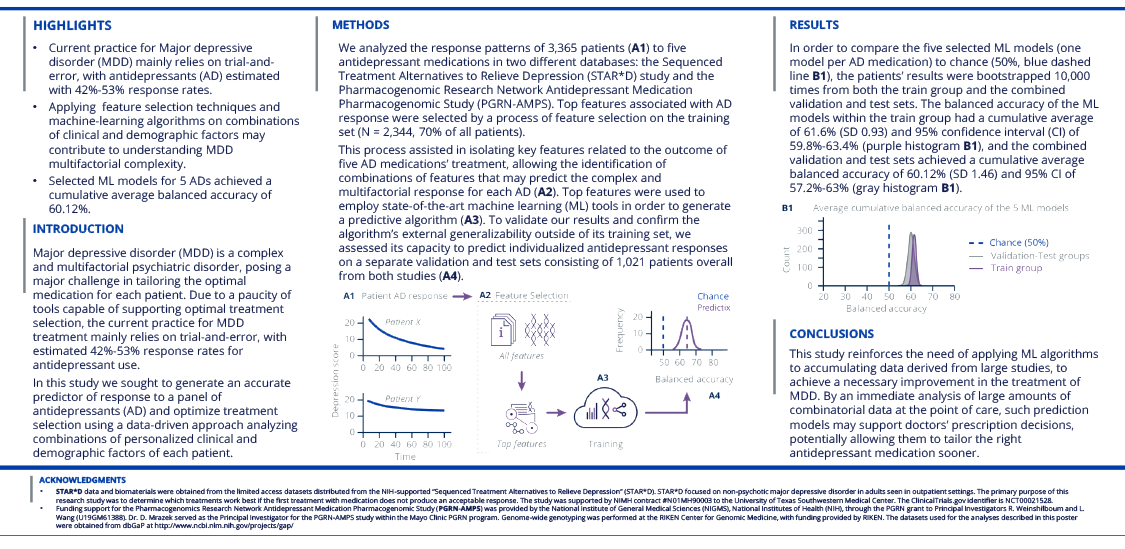
Conclusions
- These results show that using rigorous machine learning approaches and integrated clinical and demographic data can help improve prescribing accuracy.
- By analyzing large amounts of combinatorial environmental data instantly at the point of care, PREDICTIX can help support doctors’ prescription decisions, allowing them to tailor the right antidepressant medication sooner.
Hear how we helped psychiatrists around the world
“PREDICTIX is like the cutting edge of psychiatric treatment as things stand today”
“I can tell you that it seems to be perfect to guide towards the most appropriate therapeutic choice”
“…PREDICTIX is the frontier of personalized medicine in psychiatry by delivering to psychiatrists and GPs state-of-the-art information…”
Author of “Essential Psychopharmacology”
Contact Us
+972 (0)77 335 2506
2 Prof. Yehezkel Kaufmann St. Tel Aviv-Yafo, Israel
Get Started
Let's help patients recover sooner with PSY