By Dekel Taliaz
April, 2020//
The journey from gene to mental health behavior, like space, is unimaginably complex. Artificial Intelligence algorithms, like GPS tools, provide a route to map the brain’s genetic complexity to develop life-changing personalized medicine.
“For every complex problem there is an answer that is clear, simple, and wrong.” H. L. Mencken
Imagine you are at the other side of the galaxy and urgently need to get home. There are at least 500 billion planets in the Milky Way, and an estimated trillion galaxies containing 1024 stars and 1078 atoms in the visible Universe. You must choose the correct order of planets to “slingshot” your space ship home!
With an endless number of options to get the desired result, it could take you a long while!
This is a bit like understanding the brain’s role in mental illness. But, this is also, where I see Artificial Intelligence (AI) being the answer.
AI can act as our GPS to help navigate the brain space to arrive at our desired destination – to unravel its genetic complexity and assist people in coping with mental illness.
To do this, we first need to understand the long and complex road that leads from our genes to our behavior.
The complex genetic journey that results in behavior
Our behaviors are the result of complex, often non-linear, gene-gene and gene-environment interactions, combined with random processes associated with the interconnection of millions of neurons and synapses. This complexity has made progress into the genetics of mental illness exceedingly difficult.
Furthermore, despite our rapidly improving ability to quickly read and sequence a human genome, we don’t know what most of the genome actually says. We know about straight-forward genes – where a change causes a specific behavioral change in phenotype. For example, in the genetic disorder Fragile X, the mutation of the FMR 1 gene on the X chromosome is directly correlated with mild to moderate intellectual disability.
However most of the time – it is much more complex and interconnected then this! Understanding the role of genetics in depression is an excellent example of this complexity.
Artificial Intelligence (AI) algorithms and Big Data approaches can allow us to unravel these genetic mysteries and place them in the environmental and clinical context, to provide a new approach to treat mental illness.
This thinking was the basis for establishing Taliaz. We use AI scientific expertise to map the complex genetic journey involved in mental illness to develop an AI prediction algorithm that can help personalize treatment.
We have made a start with depression. Our user-friendly tool empowers doctors to match patients with the most effective antidepressant based on their genetic, environmental and clinical background. As the role of the doctor is key in mental illness, our approach is to assist them. To give them an AI-based GPS tool that helps them better predict the “right” antidepressant for each and every patient.
The Taliaz AI solution is easy to use but it is based on a complex scientific model. I will try to highlight the main points.
The cascade of interconnected signals resulting in behavior
To provide a new treatment approach using AI algorithms for mental illness, we need to first understand the signaling pathways associated with different behaviors. We need to understand the complexity of genetics – the number of genes involved as well as the cause and effect of each and every gene-gene interaction.
An excellent example of these genetic complexities can be seen during my PhD studying the role of brain-derived neurotrophic factor (BDNF) in depression.
We showed that knocking down the expression of the BDNF gene in the hippocampus resulted in depressive-like behaviors in animal models. We provided direct evidence that actual low BDNF in specific brain sites induced depressive-like behaviors and affected neurogenesis (ability of neurons to regenerate at the adult brain). However, while trying to translate these findings into the clinical world, we realized that these findings were only part of the whole picture.
Why?
The gene story is much more complex. We realized BDNF is only one gene within a cascade of genes – with each gene being able to interact and effect each other. This is seen in the complex BDNF gene cascade below.
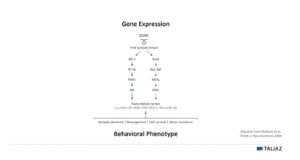
Why is this important?
To find new treatments for depression we need to look at the complete picture. We need to delve much deeper into understanding each cascade and each gene’s interconnectedness that leads to a resulting behavioral phenotype.
This is where AI comes in.
Using AI to make sense of genetic complexities
The role of models, big data, data scientist acumen and algorithms in the quest for valuable insight and meaningful results is never ending and transforming healthcare.
However, to build an AI algorithm that can effectively predict which is the right treatment for each individual is a complex process involving in-depth research.
Firstly, we must define the model. We must select the important features. We must choose and rate which gene is important based on our biological understanding and testing of different models. Basically, we must find the right models and take out the non-essential – the noise in the data. We must ‘feed’ our algorithm the best subset of features, based on our research, that are the most important predictors to achieve our desired outcome.
This is exactly the core scientific development we have done at Taliaz, by building our AI Algorithm, Predictix, to empower clinicians to choose the right antidepressant for their patients sooner.
By looking at tens of thousands of different gene combinations alongside demographic and clinical Big Data variables, our AI algorithm has helped us reduce down the number of features to those that matter.
We have built a predictive model that uses important gene, demographic and clinical combinations to give a desired performance. When the number of features, as in the case of depression, is very large and complex, this approach to selecting and weighting the features can determine whether AI succeeds or fails.
How AI will revolutionize personalized medicine in mental illness
The opportunities from AI in personalized medicine is endless.
While we can generally solve any problem, AI can help us correctly identify the problem to the solution. AI is able to unravel the brain’s complexity – to learn from each piece of data it is given and rapidly re-evaluate its analysis as more and more data is received.
So what does this mean?
For patients, we are getting ever closer to understanding the complex journey from gene to behavior, and by unraveling these mysteries, we are better able to personalize treatment. Doctors and researchers will soon have the tools to better identify problems and, subsequently, the potential solutions to those problems.
For the depression sufferer, AI algorithms, like Predictix, mean the days of trial-and-error treatment are coming to end, with doctors empowered to target antidepressants based on a person’s genetic, clinical and demographic profiling.
We have only just begun to scratch the surface of AI’s potential in healthcare, and particularly in mental health. The future looks complicatedly uncomplicated!
Join me in my next blog, where I will discuss how clinical and environmental factors can further help us crack the genetic mysteries of mental illness, but add another layer of complexity to the analysis.
__________________________________________________________________________________________
Taliaz D., Stall N, Dar DE, Zangen A. Knockdown of brain-derived neurotrophic factor in specific brain sites precipitates behaviors associated with depression and reduces neurogenesis. Mol Psychiatry. 2010 Jan;15(1):80-92.